In recent years, research on automatic human motion generation has achieved impressive results. A prime example is MoMask, a state-of-the-art model combining transformers and variational auto-encoders to create expressive animations from short text prompts—think “A person doing a karate kick with their left leg.”
But there’s a challenge: these models are usually limited to motions similar to those in their training datasets. While large datasets of motions exist, e.g. HumanML3D with 14'616 annotated motions, the manual text annotations often lack necessary detail. Further, some are high-level (“a person dancing waltz”), while others are more detailed (“a person lifts their left leg to the side”).
How Can LLMs Help?#
Manually refining these annotations would be very labour-intensive and impractical for new datasets. To bridge this gap, we developed a text refinement pipeline based on Large Language Models (LLMs). Our approach can enhance motion generation by:
- Adding contextually relevant details to motion descriptions.
- Converting high-level descriptions into structured, low-level motion sequences.
The second point is crucial—by tapping into the vast world knowledge of LLMs, we enable Text2Motion models to animate actions they’ve never explicitly seen in training. This is particularly valuable for complex motions like yoga poses, dance styles, and niche sports movements. For example, our dataset lacked examples of a “broad jump”, which can lead a model to default to similar motions, like jumping jacks. By refining the text description, we provide the model with clearer guidance, resulting in more accurate outputs:
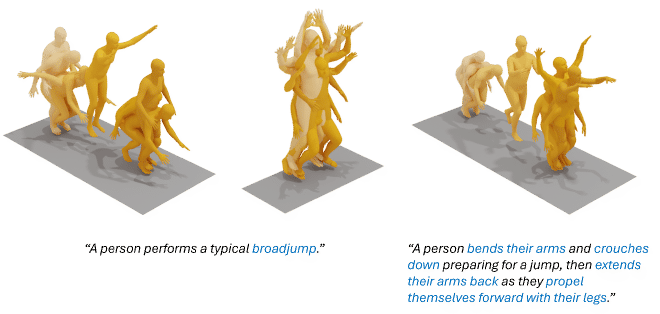
Setup#
We tested our pipeline with OpenAI’s GPT-3.5 Turbo and a locally deployed Llama3 8B model. GPT-3.5 performed better across all metrics (R precision, Fréchet Inception Distance, Multi-Modality) and respected structural constraints given by the system prompt better. Further, we fine-tuned MoMask using our refined dataset. If you want to test our pipeline yourself, we open-sourced all necessary code and provide instructions on GitHub.
Shortcomings of Text Refinement#
While we observed improvements for motions not contained in the training set, our LLM-based text refinement is far from perfect. We encountered:
- Hallucinated details—the LLM may invent motion elements, especially for simple motions.
- Omitted information—key details, like movement direction, were sometimes lost.
- Amplification of inconsistencies—Our refinement can amplify existing labelling inconsistencies.
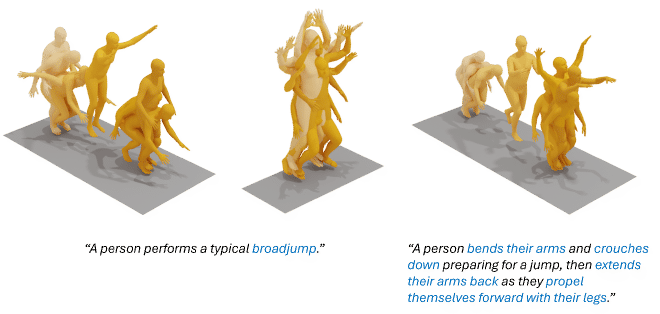