Click here to check out the academic publication associated with this project
Virtual reality training for large, industrial machines—think of it like a flight simulator, but instead, you train to operate a multi-million dollar industrial machine—offers many benefits. They provide a safe and controlled learning environment, reduce costs as no expensive equipment can be damaged by trainees or machine time has to be provided, as well as easy global scaling as all you need is a VR headset. The ETH Spin-Off AtlasVR is working on unlocking exactly this potential.
However, creating such virtual training experiences currently is a time-consuming, manual process for them and requires expert knowledge in game engines and visualization. To make things worse, the responsible programmer usually is not an expert on the industrial machine, resulting in a communication overhead for exactly specifying the training scenario and design iterations. The solution? An authoring toolkit offering a simple method for creating, testing and distributing customized training lessons. This was the topic of my industrial Bachelor’s Thesis together with AtlasVR and ETH’s Innovation Center Virtual Reality (ICVR).
Authoring-By-Doing#
The final toolkit incorporates an “Authoring-by-Doing” approach, as proposed by Wolfartsberger & Niedermayr in their paper. Instead of coding complex training sequences, machine experts can simply record themselves interacting with a 3D version of the industrial machine inside VR. Actions are captured in real-time, recordings can be segmented into steps and lessons and voice-based narration can be added for further clarity.
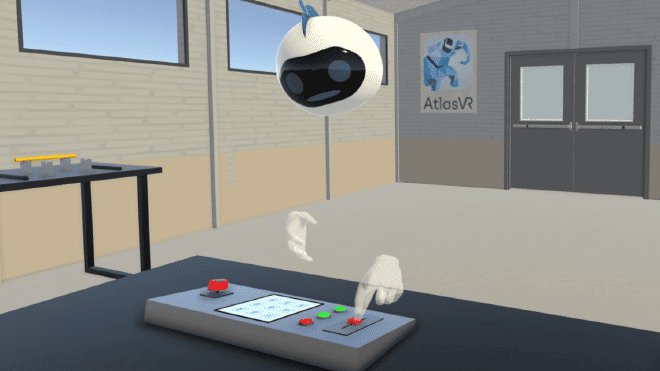
Trainees can then watch these 3D recordings inside VR, using standard playback controls. Unlike traditional video tutorials, VR recordings allow users to view the expert’s demonstration from the exact same perspective, or any custom angle. Difficult steps can be replayed as many times as needed, allowing trainees to reinforce their understanding while simultaneously interacting with the digital machine.
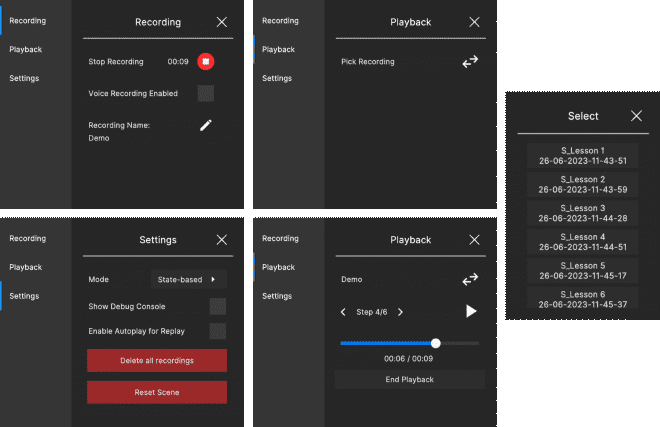
Recorded content can be saved, loaded, and exported to common formats, such as CSV and JSON, for sharing and analysis.
Comparison of 3D Recording Approaches#
While VR authoring toolkits are still rare, my research aimed to address an additional key gap:
How should 3D recordings be implemented for the best memory footprint, performance and accuracy?
To explore this, I implemented two distinct recording approaches:
- State-Based Recording: Captures the position and orientation (6 degrees of freedom) of both the expert and all objects at every moment in time. To optimize memory usage, only changes are stored.
- Input-Based Recording: Instead of continuously saving object states, this method only records the expert’s interactions (e.g., button presses, hand movements). When replaying, the system reconstructs the behaviour of the digital machine and all other objects by resimulating interactions.
Thus, I implemented the Authoring Toolkit’s recording functionality with two distinct approaches, namely a state-based and an input-based approach. The state-based approach involves capturing all 6 degrees of freedom of the user and all objects at any point in time. A simple optimization I performed was only capturing changes, which heavily reduced the memory footprint. The input-based approach aims to even further reduce the memory footprint by only capturing the user’s interaction. This subsequently requires a resimulation of interactions when replaying a 3D recording.
I quantitatively compared these two approaches inside a virtual testing environment, covering a broad range of interactions, based on three performance indicators:
- Memory Footprint
- Performance Overhead
- Replay accuracy
If you are curious about the results, check out my corresponding paper.